THE ECONOMIC POTENTIAL GROWTH MANAGEMENT FOR REAL ESTATE DEVELOPMENT COMPANY THROUGH AUTOMATION AND ARTIFICIAL INTELLIGENCE TECHNOLOGIES
DOI:
https://doi.org/10.36690/2674-5208-2023-3-99-114Keywords:
economic potential, potential growth, real estate development company, development management, primary real estate price, fuzzy logic multifactor model, management automation, artificial intelligence technologies, informed management decisions, digitalizationAbstract
Constant changes in the economic environment of development companies, as well as a large number of impact factors on the economic results of these companies, complicate the management processes of their economic potential formation and growth. Optimization of the management system for the economic potential growth of development companies requires the implementation of multifactorial models of informed decision-making, built on algorithms of fuzzy logical inference. Based on this, the article develops a multifactorial input-output model of fuzzy logical inference regarding the primary real estate price of the development project. The model is dynamic because it includes the time impact factors on the resulting values, thus it can be used throughout the entire cycle of the development project implementation. It grants obtaining of two output price values: full and with the maximum possible discount, depending on the input data for a specific real estate object, which is a tool both for increasing the company's pricing policy management effectiveness, as well as for developing of a system for profitability maximization of each development project. In addition, the algorithm of this model application in the economic potential growth management system of the development company is proposed, as well as the spheres of its practical utilization at different levels of managerial decision-making are considered. Analysis of the developed model disadvantages led to the development of methods for their minimization through automation and artificial intelligence technologies. The application of the Simulink environment of the MATLAB software complex made it possible to develop a software implementation of the developed model, which allows not only to minimize the identified disadvantages but also to apply artificial intelligence technologies for its further improvement. The software implementation, taking into account the features of the model, is presented in the form of a hierarchical system consisting of three subsystems, each of which is not only a component mechanism for obtaining the model resulting values but also allows for obtaining intermediate values for each separate subsystem, which favor multi-level result analysis to make informed management decisions.
Downloads
References
Aghion, P., Jones, B. F., & Jones, C. I. (2018). Artificial intelligence and economic growth. The economics of artificial intelligence: An agenda, 237-282.
Akselrod, R., Shpakov, A., & Ryzhakova, G. (2022). Economic and management predictors of the transformation of operating systems for construction development in the context of digitalization of the economy. Market relations development in Ukraine, 12(247), 113–121.
Bielienkova, O. (2019). Klasternyi analiz rynku yak analitychna komponenta vyboru konkurentnoi polityky developera. Actual problems of economics, 6(216), 141-158.
Bielienkova, O. (2020). Vdoskonalennia mekhanizmu upravlinnia konkurentnym potentsialom developera zasobamy shtuchnoho intelektu. Actual problems of economics, 3, 107-122.
Bolila, N. (2022, March). Zaprovadzhennia tsyfrovykh tekhnolohii upravlinnia budivelnymy pidpryiemstvamy. In Proceedings of the international conference Aktualni problemy suchasnoho biznesu: oblikovo-finansovyi ta upravlinskyi aspekty (pp. 164-166).
Bresnahan, T. (2021). Artificial intelligence technologies and aggregate growth prospects. Prospects for Economic Growth in the United States. 132-170.
Ćetković, J., Lakić, S., Lazarevska, M., Žarković, M., Vujošević, S., Cvijović, J., & Gogić, M. (2018). Assessment of the real estate market value in the European market by artificial neural networks application. Complexity, 2018.
Guan, J., Shi, D., Zurada, J. M., & Levitan, A. S. (2014). Analyzing massive data sets: an adaptive fuzzy neural approach for prediction, with a real estate illustration. Journal of organizational computing and electronic commerce, 24(1), 94-112.
Niu, J., & Niu, P. (2019, December). An intelligent automatic valuation system for real estate based on machine learning. In Proceedings of the international conference on artificial intelligence, information processing and cloud computing (pp. 1-6).
Park, B., & Bae, J. K. (2015). Using machine learning algorithms for housing price prediction: The case of Fairfax County, Virginia housing data. Expert systems with applications, 42(6), 2928-2934.
Rossini, P. (2000, January). Using expert systems and artificial intelligence for real estate forecasting. In Sixth Annual Pacific-Rim Real Estate Society Conference, Sydney, Australia (pp. 24-27).
Rosynskyi, A. (2022). Fuzzy logic inference algorithms utilization in the economic potential growth management system of the real estate development company. Shliakhy pidvyshchennia efektyvnosti budivnytstva v umovakh formuvannia rynkovykh vidnosyn, 50, 180-202.
Shaposhnikova, I. (2019). Rating modeling of the primary residential real estate market of Ukraine. Shliakhy pidvyshchennia efektyvnosti budivnytstva v umovakh formuvannia rynkovykh vidnosyn, 40, 25–35.
Shaposhnikova, I. (2020). Modern approaches to the formation of a project for the development of construction enterprises. Shliakhy pidvyshchennia efektyvnosti budivnytstva v umovakh formuvannia rynkovykh vidnosyn, 46, 126-135.
Shpakov, A., Stetsenko, S., Shpakova, H., Sorokina, L., & Akselrod, R. (2021). Assessment of the Influence of Adaptability Factors on the Effectiveness of Managing Changes in Enterprises by Fuzzy Logic. Scientific Horizons, 24(10), 72-82.
Stetsenko, S., Bolila, N., Sorokina, L., Tsyfra, T., & Molodid, O. (2020). Monitoring mechanism of resilience of the anti-crisis potential system of the construction enterprise in the long-term period. Еconomics, finance and management review, 3, 31-42.
Stetsenko, S., Tytok, V., Emelianova, O., Bielienkova, O., & Tsyfra, T. (2020). Management of Adaptation of Organizational and Economic Mechanisms of Construction to Increasing Impact of Digital Technologies on the National Economy. Journal of Reviews on Global Economic, 9, 149-164.
Stetsenko, S., Sorokina, L., Izmailova, K., Bielienkova, O., Tytok, V., & Emelianova, O. (2021). Model of a Company Competitiveness Control by Means of Artificial Intelligence Tools. International Journal of Emerging Trends in Engineering Research, 9(2), 60-65.
Strusani, D., & Houngbonon, G. V. (2019). The role of artificial intelligence in supporting development in emerging markets. EMCompass, 69.
Wang, L., Sarker, P., Alam, K., & Sumon, S. (2021). Artificial Intelligence and Economic Growth: A Theoretical Framework. Scientific Annals of Economics and Business, 68(4), 421-443.
Wang, X., Wen, J., Zhang, Y., & Wang, Y. (2014). Real estate price forecasting based on SVM optimized by PSO. Optik, 125(3), 1439-1443.
Xu, X., & Zhang, Y. (2022). Residential housing price index forecasting via neural networks. Neural Computing and Applications, 34(17), 14763-14776.
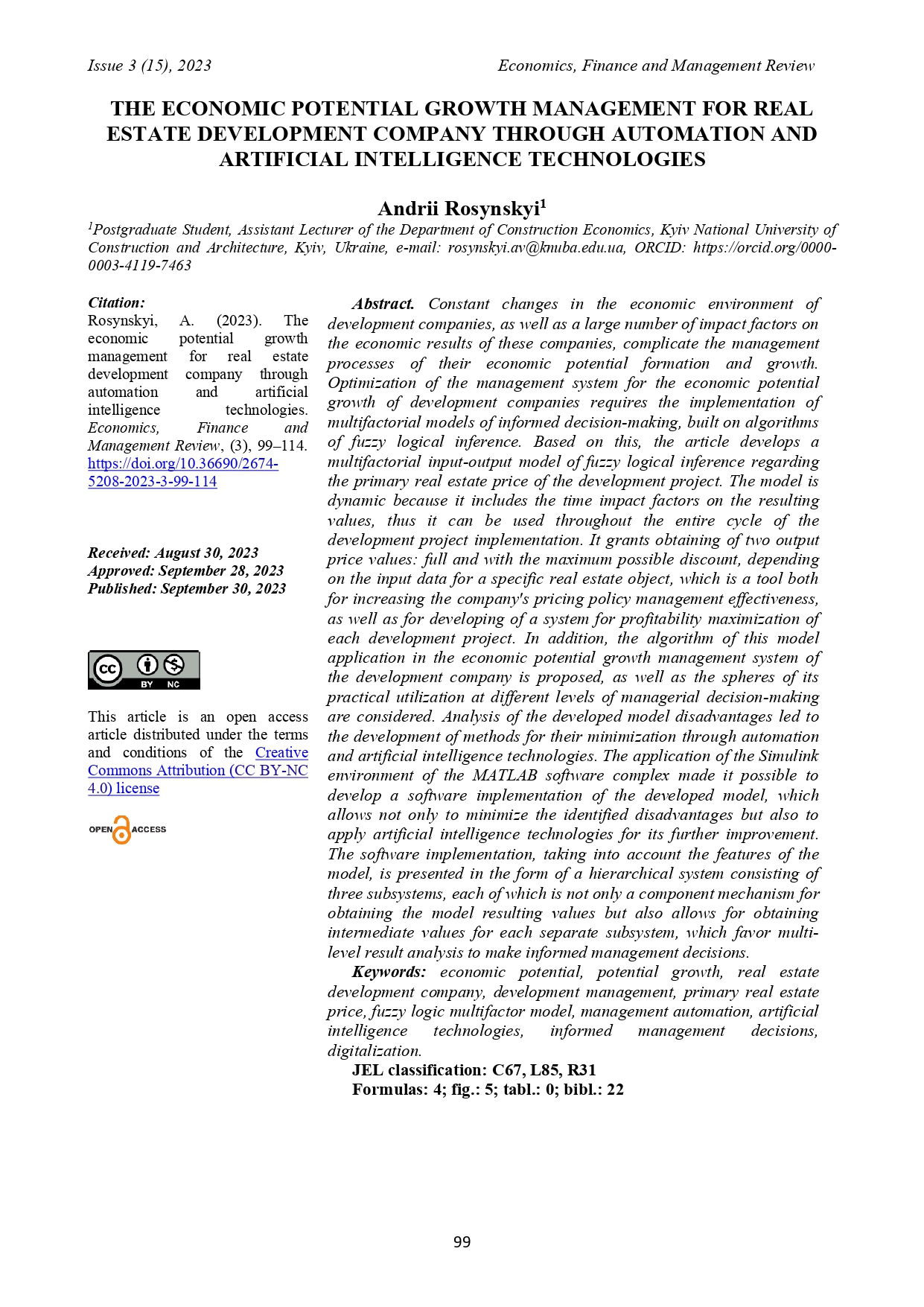
Downloads
Published
How to Cite
Issue
Section
License

This work is licensed under a Creative Commons Attribution-NonCommercial 4.0 International License.